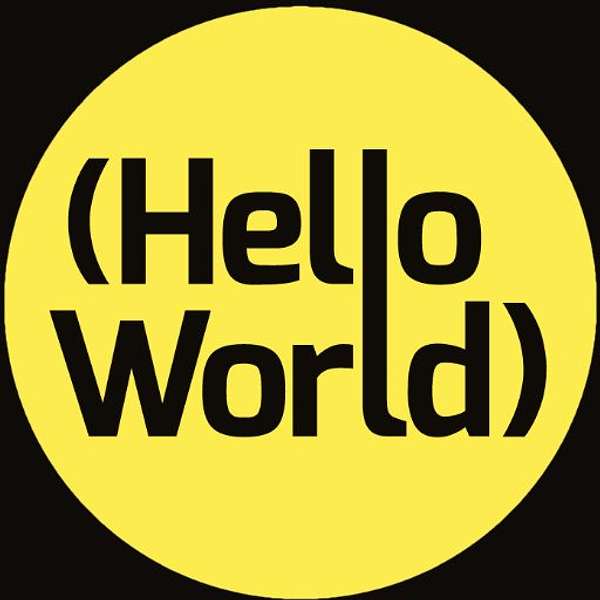
Hello World
Are you an educator who’s passionate about computing and digital making? If so, this is the podcast for you!
Join hosts from the Raspberry Pi Foundation as we chat with teachers, researchers, and learners from around the world to discuss the latest research, debate the big questions, and provide practical tips to help you engage and educate young people in computing. We’ll be releasing a new podcast series to coincide with each new issue of the Hello World Magazine to continue the conversation.
We’d love to hear from you! As we trial new episode formats, welcome additional hosts, and endeavour to produce regular episodes, we’d really appreciate your honest feedback about what you find informative, engaging, and, most importantly, helpful. Drop us an email on podcast@helloworld.cc and subscribe to the Hello World Magazine for free at http://helloworld.cc
Hello World
Machine Learning for Kids: why introduce young learners to AI?
Although artificial intelligence (AI) is a developing field, its applications are all around us and increasingly part of our everyday lives. This week, James and Carrie Anne explore why educators should introduce even their young learners to the principles of AI, and they explore some activities and experiences to develop learners' understanding. Not only are many learners likely to use or even develop AI as part of their future careers, but they should all understand the principles, benefits, and risks associated with the technology.
Full show notes:
https://helloworld.raspberrypi.org/articles/machine-learning-for-kids-why-introduce-young-learners-to-ai
It's about understanding how the
Carrie Anne Philbin:When and how do you introduce
James Robinson:Machine Learning isn't this It's here, it's here, it's now we're using it.
Nic Hughes:And a lot of kids often ask, how What is going on?
Carrie Anne Philbin:You should let young people play Welcome to Hello World, a podcast for educators interested in computing and digital making. I'm Carrie Anne Philbin, a computing educator, content creator, and it doesn't matter how many jokes you train me with, I'm still not very funny.
James Robinson:And I'm James Robinson a I work on projects promoting effective pedagogy within our subject. If you want to support our show and subscribe wherever you get your podcasts, and please leave us a five star review.
Carrie Anne Philbin:Today, we'll be exploring an education and asking what is machine learning? When and why should we be teaching it? We're going to be joined by some great guests, to bring us their perspectives on this topic. But before we hear from them, I thought I'd get your perspective, James. How would you describe machine learning and AI and why should educators be teaching it, in your opinion?
James Robinson:It's a really interesting and as that emerging kind of topic is something that we've touched upon this in a previous episode of the podcast, that, that machine learning and AI kind of surrounds us in many ways, there are so many facets of our life which utilise machine learning technology, that our young people having an awareness and understanding of what it is, how it works and what its implications are, I think is really important. It's one of the next big kind of areas for them to come to grips with and to grasp, I think, to describe what it is. I think we see machine learning all over the place, but it's where we we are sort of outsourcing some of our intelligence to the computer. We're training it, we're providing models. We are giving it lots of prior experience to then make decisions based upon. And it may not will be making informed decisions in the way that we make informed decisions like weighing up the pros and cons necessarily. It may be spotting patterns and and looking for sort of similarities between things. And those patterns may not be super apparent to us as human beings. Looking on the outside, the machine may be making judgements about patterns that we can't see. So it's really super fascinating as an area we see in things like we've mention our Netflix recommendations in the past, we see machine learning, having applications, autonomous vehicles is quite often a frequently cited example. And I think within the curriculum, certainly within the within the English curriculum, there's definitely space for this is not something that's explicitly mentioned in the curriculum. As you know, we must study machine learning, but there is definitely scope for looking at future developments and emerging technology within the aims of our curriculum. So I think it's definitely something that educators should be to be alive to and understanding and thinking about how and when they should be introducing it.
Carrie Anne Philbin:Yeah, I think what you're kind about when I think about digital literacy. I hear this terminology used all the time that that we should all, whether we are adults or children, be digital, digitally literate. Right. So we should understand the technology that is around us. For me, that's not just I can move a mouse or push a button or write a word document. It's "do I understand the technology that is ubiquitous around me?" And you've you've cited some really good examples there, like Netflix use there. But I would even talk about social media. You know, we scroll through social media and social media recommends certain things for us to understand and content we might be interested in. Right. But the more that that happens, the more we create a silo to ourselves. And that has actually wider implications. And we've seen that with elections over the past few years in different countries. I think there's something about the large scale collection of data that happens for all of us as we use data that's being tracked about our movements and what we do and what we eat, all of that. I think we should know a little bit more about what happens to that data. I think machine translation is something I've been really interested in over the past few years. We can use tools to help us translate words into different spoken languages. And that's really great, because it helps connect us around the world. But sometimes the machine isn't. I mean, it has been trained well enough or perhaps isn't clever enough, I hate to use that phrase, but it doesn't ensure where there are languages with different genders. But there is always they don't accidentally always default into a male language. Right. Masculine verbs and so on. So there's something about for me about digital literacy, which means we should all whether we are a teacher or not, we should all care about that, right?
James Robinson:Yeah, absolutely. And then for think that about how do we delve deeper to those that do have that passion can really start to understand the nitty gritty and start to be the developers of machine learning and AI of the future.
Carrie Anne Philbin:I'm glad you're kind of said maybe we were getting a bit too niche. Because you and I are colossal nerds. But now I feel kind of reassured that this is a good topic. And I'm certain that our guests are going to help us with this. It's pretty clear we're going to need some help in exploring these questions. So I think we should probably get them involved.
James Robinson:Let's do that yeah.
Carrie Anne Philbin:So joining us is software expert and author of the"Machine Learning for Kids" resources, a collection of project based activities to support young learners in developing their understanding of machine learning. Dale Lane. Welcome, Dale. What is machine learning and why should we all know more about it? Did we get it right?
Dale Lane:Yeah. Yeah. Yeah. Spot on machine learning is an alternative approach to getting our computers do something. Instead of us giving the computer instructions that it should just follow step by step, with machine learning, we get computers to learn how to do a task by by learning from data. We give it examples, it learns how to reproduce that. As for why, why it's important for children to learn about it. I think, like you said, it's about I mean, you described those digital digital literacy, but I think the more general point is it's it's about understanding how the world around them works. Because I often when I talk to friends and family who are not in the tech sector, they sort of have this assumption that AI and machine learning is future sci-fi and something we'll do when we have spaceships and live on Mars. And it's to do with robots. And like they don't associate things like Siri and Alexa as being AI, they don't think of when like the Smart assistants in our car as being AI. And often they're quite surprised when you point out that A.I. is here today and we all use these systems all the time. And, you know, children are naturally curious and want to know how the world around them works. And I think explaining to them the basics of what machine learning is, is a really key part of that.
James Robinson:I think you've reminded me of favourite speakers, Simon Peyton- Jones, said to me in an interview I was doing with him a little while ago, where he said, you know, we we teach our students all about the physical, the chemical and the biological realms of nature. And, OK, this is an artificial kind of construct, but it's still the world around them. And it's, you know, why would we not educate them about all facets of that? And as you say, you know, machine learning isn't this futuristic technology. It's you know, it's here. It's now we're using it for better or worse. So so we should understand i and it's implications.
Carrie Anne Philbin:So what are the key principles learning that you think kids are curious about?
Dale Lane:I guess the two sides to it are And I think because how often the explanation Kids see about AI and machine learning is what they see in the media, which has a mixed history in accurately describing what the tech can do. So I think part of it is just that baseline idea of what is possible today and what isn't. I think the other side that I found that children get really engaged in is, is that what I would think of as a debate about AI and ethics, about what should we be doing, what's appropriate, what is fair? Even at quite young ages that students seem quite up for engaging in that debate about what they think is a reasonable use of AI and not just the use of AI, but use of their data. I mean, you mentioned before that so much data is being collected about everything we do and what we buy, what we watch, what we listen to, who we talk to. And all of this is being used in machine learning systems. And I think the next step, after helping them recognise what these systems can do and what they're being used for is getting them to start to think critically about what they think about that. And because I think that's a debate that society needs to engage in. I think we've been quite slow to allowing tech companies to sort of do whatever they feel is cool and shiny and that there's a need for us to catch up as a society and have a debate about what we're comfortable with and what we think is appropriate. So starting at the education level, starting having that debate in the classroom, getting students to start thinking about these topics is a really good way of seeding that.
James Robinson:And what kind of conversations learners in your experience, when you do ask them to kind of debate that the ethical or moral or the or the use cases for machine learning, what kind of ideas and challenges that they kind of perceive with its use?
Dale Lane:There are lots of different be sort of bias, this idea that we sort of assume that a smart computer is going to give us the right answer and a poorly coded, poor algorithms, a dumb AI will give us the wrong answer. But once students start to play with this stuff and start training their own models and they start to see that the computer learns from the examples we give it, it learns. It picks up on patterns in the data that we choose to give it. And it's actually the point that you were mentioning earlier about translation systems, that the computers aren't smart enough to recognise gender defaults or genders and language. It's not that the algorithms aren't smarts enough. It's that they're reflecting back to us the biases our own society and our culture. We fed them with a lot of documents that have these assumptions that doctors are male, that nurse is a female. And then we're surprised when actually it's learnt from us. It's spotted those patterns and it's reflecting them back to us. So I think it's actually those kinds of experiences are really great for students to actually start to question how we want these systems to behave, because then you can get into debates about, well, should we be sort of skewing, influencing the answers the system gives and not just reflect the training we've given it, but actually if we want to start influencing it so that it behaves more the way we think it should do. So actually, the gender in health care is a really nice example. If and actually the other example, as I've seen a lot of CEOs I've seen examples of. Of systems where they assume a CEO is a white, middle aged guy in a suit. If you just collect examples and give back, then that is what it will learn. So what responsibility do people who are creating the systems have, not just for blindly collecting training data and then thinking that absolves them of any responsibility, but actually thinking about how these systems are going to be used and the outputs of these systems and what they have to do to to make sure that the output reflects what it what it should.
Carrie Anne Philbin:So Dale has been talking a learners kind of interact with this and start to to learn more about machine learning. And thankfully, we're joined by Nic Hughes, who is a teacher and computing consultant who's recently been teaching his learners about machine learning and AI. What age group of young people do you predominantly teach? And what was your motivation for introducing them to machine learning?
Nic Hughes:So I mainly teach key stage two I've only ever really done the machine learning with the top end of that. So probably year five and six children. Why did I start trying to do it? I think after seeing it demonstrated, I met Dale at a Raspberry Jam, I believe it was. I think you would demoing at an event there. And I was quite amazed by what it could do. And I think some of the questions it started to open up, which is what we've just been talking about to do with digital understanding and bias in data. And all of these types of questions opened up loads of questions. And the children in our classrooms today do do we interact with AI all the time. As we've said, they've got these smart speakers or they've got Siri or they've got Alexa, all this sort of stuff. So the idea to try and start and to get children to understand how they work, I just seem to be a very logical thing to start talking about. And a lot of kids often ask like, how does that work? What is going on? The really inquisitive children will ask that. And it isn't as simple as"oh it's programmed" because it isn't programmed quite in the same way. And so suddenly opened up this new sort of area to talk about. And of course, I hadn't really heard a lot about machine learning before seeing Dale talk about his great resources from that I've been so interested in over the last few years. And I think it's a real important area to start exposing our children to as early as possible. I think the moment the moment they start asking questions about bias in media, and I think it's a big question. We talk about from a digital literacy point of view all the time with our older children in primary. It's important to start thinking about that from the data sets we're starting to collect and what does that mean? And all of those questions which we've already been talking about are all questions I've seen children ask me about how does this work and what how how does this work. And Google Photos is a great one, that's the thing that was quite fascinating to me. I think that's the example that I don't think anyone mentioned. That, of course, Google Photos is based on machine learning and the fact that how does it know that what the pictures are? Someone told it. And that's fascinating little experiment just to show."Oh, let's just type this in, and look at all the photos it's picked up. So I've never tagged it Minecraft, but it shows me the Minecraft pictures in my library straight away because it just knows it is quite fascinating to kids to see that.
James Robinson:It's when it starts naming and it's like, oh, "that's your mum", and I'm like, "what?", you know? And I think it named one of my siblings by a nickname like I was like, "how did you? What?" I don't know where that came from. No, that's fascinating. I think, you know, some people might be listening to this and thinking that's quite brave to kind of try that experience with such young learners. I'd be interested in your reflections upon that experience, like were there aspects that they really took to were there aspects that they found challenging and were maybe a bit too soon for them? But what's your kind of reflection on that experience?
Nic Hughes:Sometimes the programming took looking at making the data set. So there's some really nice examples on there. One of the examples which I use of use quite a few times is making a machine that identifies the meaning of text, like is it not nasty or nice? That's the really simple idea. But it was creating the dataset for that, that I think it was the most interesting part for the children to work out the oh, you know, you have to give it loads of examples. And then from the examples set, you have to train the machine and then. It might work, it might not work, and I think the idea of you inadvertently build bias into your data set accidentally, especially when the kids were generating their data set themselves. That was just an interesting conversation to have. So the programming side of it probably less so because I know we're talking about it's not Programming. There's still an element of you've got a start within the tools that we the on the website your structuring it using scratch or a bake of scratch, isn't it, with the machine learning back in the, built in there. But you've got to structure sort of the the algorithm, I suppose it's following, but then of course, it's pulling in the data set. So yeah, it was that that was the side that I think was really useful. The kids were getting the idea of what the data set is doing, things that we're struggling with. I think sometimes it's those wider questions, some of that that the ethics side, I think, depending on their age. That's an interesting conversation. We did talk about the ethics of cars and how does a car know who to kill, which is a horrible thing to talk about. But that was a question that came up. It was like, how does it you know, it is a really old ones do, but it was that's a quite a struggle to work through because you go and the kids are very interesting views on which ones it should be, which is like, okay, whatever. But but that's the sort of thing I'm like, how to keep things safe and what what those things are. And there's a tool to do that isn't there there's a training tool for that I've seen online, which we did look at and explore a little bit because the kids are sort of interested in it. But it's that real high level ethics. I think some of it is is difficult for some children.
Carrie Anne Philbin:Not who to kill, who to save!
Nic Hughes:Yeah, it's save. Yes. Yes. Yeah. Well, yeah, yeah. It's true actually it's one way or the other, isn't it? Yeah. Yeah. The computer has to decide who to save. Yeah, that's accurate. But yeah. So yeah. Is is that side. Yeah.
Carrie Anne Philbin:And if people remember back to actually spent a lot of time talking about the trolley problem and about ethics and how to have classroom discussions around ethics, probably much older children. So it's really interesting to hear your view on kind of how that lands with younger children. And but we've spent a lot of time talking about machine learning for kids the tool. So perhaps Dale you could explain to us what is machine learning for kids and how can educators use it?
Dale Lane:So there's a few different On the one hand, it is an online Web based way of training, a variety of different types of machine learning models with a an interface that I tried to make as simple and child friendly as possible. So children can prepare data and anything they can think of. It's, either collecting sound recordings or pictures or sets of numbers or words or whatever it is, and then use that to train their own custom private machine learning model to do whatever it is that they've thought off. The second element is, as Nic sort of mentioning, integration with educational coding environments. So Scratch was the first one I did and the one that's used the most. And there was a couple of reasons for that. Partly it was so that students could use that machine learning model in an environment they're already familiar and comfortable with. But also, I think it tells a real story that similar to what Nic saying about machine learning, isn't replacing coding like the fact that machine learning is on the rise or the use of it is on the rise does not mean we don't need to learn how to code. It's just another tool in our toolbox it complements coding. It means we can do more interesting, powerful things with coding. It's not we use it instead of coding. So I think having it in scratch as opposed to something new and different helped me tell that sort of part of the story. And finally, it's a set of worksheets to help inspire coding groups and classes of what is possible. Each, as many of the worksheets as possible are based on real world uses of AI. So the idea is that students can follow some step by step instructions to make a simplified version of some real world AI application.
Carrie Anne Philbin:I actually used one of the I was playing with noughts and crosses or tic tac toe on machine learning for kids. I actually use Python to do it. And I think what is really interesting, and an aspect we haven't talked about is training an AI to ultimately beat you. What I learnt very quickly was the noughts and crosses AI was very, very quick to work out... You know after playing about ten games was very quick to start beating me, like pretty much almost immediately. And that's always super interesting, I think, for young people, do they respond to that that kind of way-in in your experience, Nic?
Nic Hughes:No, it's interesting. Actually, teaching of programming. I think that's a method or theme that I use in quite a lot of it, but actually for machine learning it isn't. I've spent quite most of the stuff. I've worked with the kids, these things along the lines of a smart speaker or responding to something. So evither making it do something or responding to like if you say something, it knows if you're being nice or nasty. I've sort of focused on those types of examples. I know there are examples on Dale's site which I've always looked at, but I've never felt the need to go quite as far as that. And maybe that's because we're, when you start introducing gaming, there's suddenly a lot more complexity to the programs that you're introducing, selection and you're introducing variables and things of that. So to some children that that's enough complexity without also adding on that machine learning level as well and that new data set. So it's probably something that I've it it sort of sits above but also sits below that in terms of the complexity of how you might want to try and teach the children, I think it all depends on your cohort of kids, to be fair, if you've got some really keen ones. I'm sure it's a great thing to do to extend stuff, because, of course, there's plenty of games that we make, You could easily get them to get the machine learning model to to beat you at it. So, for example, we've I've made plenty of space invader or Pacman type games over the years, which, of course, it's ideally suited to do that. I've seen some examples of that, but I've never pushed quite as far as that a primary into the gaming side.
Carrie Anne Philbin:I think one of the great things decision tree. I think I really liked about about doing this was training it, training the model, and then being able to go back to machine learning for kids and see the decision tree that had been built by playing the game over and over. And that was really interesting. But I also in scratch a game I created was a rock, paper, scissors. I think using Google teachable machine very quickly, using that tool and then embedding that in scratch is another really great way for younger children to start thinking about, like how many images do you need and what kind of images will represent the the different gestures and programming those, I think, is a really nice, nice way into. James?
James Robinson:I think that just to Nick's with with the early students, maybe to go, or wanting to go into the sort of more advanced programming things, I think one of the things that plays really nicely primary, in my opinion, is that a lot of what machine learning is doing is about categorisation. We're taking things and we're categorised them as naughty or nice or, you know, cat and dog. And I think that that's something that in their formative years of education, pupils spend a lot of time kind of categorising and cataloguing and identifying things. And so that that experience is almost sort of second nature to them so it plays really well, I think, to that. And then your mention Carrie Anne of the decision tree reminds me of a really nice unplugged activity. And I'll have to find out who did it. I think it might have been Paul Curzon. I'll find a link to it, where you're teaching a sort of machine to play a game and you're using little sweets to reinforce. You got little sweetie cups. And every time it makes the right decision, you top up that cup. So that's... So you can kind of reinforce the most appropriate decision at that moment. And that's a really nice way of getting it across. Away from the computer and, away from the programming. That's really interesting reflection, I think when you when you've got that sort of sandbox toolkit environment and, you know, you can just make observations and spot things and learn things that you just you didn't think you were going to or that. Yeah, and you can go as nice kind of happenstance kind of moments, Carrie Anne?
Carrie Anne Philbin:As ever play is useful in Who knew? Who knew we should let young people play and tinker.
James Robinson:Absolutely. So, I've got a actually. So we've talked about and we've mentioned quite a few different activities. But if we were to think about this sort of suite of activities that are there and it could be from from Dale's set of resources, or it could be from the wider kind of resources that are available. What's your favourite activity to really kind of engage learners or demonstrate the power of machine learning or get them discussing something? What would be your recommendation?
Dale Lane:You mentioned the Rock Paper happened once, and it was a total fluke but I've told the story a few times because I really like it. I was with some, so for people who haven't seen the rock paper scissors project. The idea is that you use the webcam, you hold your hand up to the webcam to make different shapes. The computer learns what a rock looks like, scissors and paper, and then it plays against you. And it was I think it was like the second or third time I've run this with the class. And there was a student who did a really good job of collecting examples of the hand, doing rock and scissors. And then when they were taking pictures of their hands for paper, it so happened that one of their classmates had come and stood next to them and sort just about in shot and was in the picture while they were taking their photos and then went back to finish their work. And what they hadn't spotted was they'd trained the machine learning model to think that a hand, meant rock or scissors and that a hand and a person meant paper. And they hadn't noticed they'd done that. And it was only when they'd made that scratch programme and they'd started playing it and it was getting rock and scissors. And then the teacher or I would come and stand next to them over the shoulder, see how they're getting on. And it kept saying paper, no matter what they did with their hand. And they thought it was broken. They thought there was a bug. They thought was something wrong with that code. And we went back to their examples and said, well, we'll do all of these have in common. What have you taught the computer? The computer didn't know the hand was the important bit. The computer learnt what you taught it and you taught it that a person meant paper. And so what I love about I mean, it's been ages since I've been in a classroom because obviously Covid. But what I used to love most about the projects, was giving them time to explore and experiment and stumble onto those kinds of lessons that I never even planned. But they just sort of accidentally through getting that chance to play in a friendly sandbox, would start to learn things about the technology
James Robinson:And tell it's like picking Which which of your activities do you think is your favourite?
Nic Hughes:So I would probably pick the devices? Is that still a smart speaker one? Yeah. You know the one way you can turn on lights and turn them fans, I think, or pick that just because it's really within kids' experiences. They know what they're trying to do there. I think I would definitely pick that one. That would be my first port of call.
Dale Lane:There's one from Mozilla called which was it came out of when there was news stories about bias in hiring practises, where a company had automated sifting through CVs, and picking who they wanted to recruit. And that ended up reflecting bias of their previous hiring practises. And it was horrendous. And what Mozilla have done is a really nice project where you can go online and you play this game where basically you decide whether to accept or reject a candidate and you're training a model. And then it you end up, it shows you the biases in what you've trained. And actually you end up without realising training, a model that is biased, and I like that because that's too easy to fall into this trap of thinking that that training a biased means you've been negligent or malicious or you're a bad person. And actually realising that it happens accidentally, the people that mean it to happen and the game forces you to do it by putting you under time pressure, it makes you make decisions quickly, quickly, quickly, and then it shows you that you've made this horribly biased system that is rejecting people for no good reason. And I've had really good discussions with classes after sort of playing through that game and talking about. But this isn't a totally abstract idea, this really happened. And and come back to what I was saying earlier about it gives you a chance to have a discussion about the responsibility that people who are making stuff with this technology has. Those are the projects that enable that ethical debate, the discussion about how things work in society. Those are the ones I really enjoy because and and I love the lessons where I've had the time to you know, once the project is finished, for us to get around and discuss it and see what their opinions are like. That is the most exciting, interesting part, the lesson, the making, that is just what enabled that discussion to happen.
Carrie Anne Philbin:And hopefully they can connect scenarios, right? There's something about making that concrete, that learning and connecting it to the world around them, bringing them back to that starting point, I think is really valuable.
James Robinson:I love that. I think that that when I was in the classroom, one of my favourite kind of things is when you do an activity. And then actually if you carve out some space to discuss and reflect and talk about whether it's ethical issues or how you approach the problem or whatever, I think that time to engage, you really get a chance to observe what the students really understand and feel about that topic.
Nic Hughes:It's just it sounds similar to resource from code.org called oceans, which I quite like. And that's that feels really primary and I think it's a really nice tool to really showcase the real basics of it, the real starting point. So that's an activity that I probably would use fairly early on with children as well.
Dale Lane:It's about picking a topic that grown-up world. The default go to project for training a machine learning model is something like a spam filter. But that doesn't work in a primary school because kids don't get enough email to know or care what spam is. So I think you're right, that, you know, would a young primary school child know what a CV is, know what applying for a job feels like. Would they feel invested and really care about how job applicants are treated? So I think yeah. And maybe oceans is a really nice example because kids do care about the sea life. And so, yeah, I think maybe picking themes that are relevant is the important bit, and maybe that is more of a secondary school sort of topic.
Carrie Anne Philbin:So it doesn't seem like age is even understanding it. The barrier is more around the context, making sure the context is age appropriate. So having some that are more towards the younger age group, kind of five to sort of seven, there's probably a context that works around marine life is probably one that's kind of good for seven to 11 year olds, maybe around smart speakers and connecting it to technology. And then probably when you get to 11 to 14 year olds, you're probably more moving towards some of those game based activities that we talked about.
Dale Lane:I do the same projects with different things out of it, like the one Nic mentioned around sentiment analysis. If I do that with a young primary school class, it's just about, bringing to their attention, computers can learn to recognise tone and sentiments, and computers can recognise positive happy text versus negative text. But I'll do the same project for the secondary school class, and we will try to see... We'll push the boundaries, like show what, like computers are really bad at spotting sarcasm or negation or, you know, actually start because you can have a debate with them about. Well, hang on a minute. If if the message is computers can recognise insults, then why is trolling on social media still a problem? Why hasn't it been stamped out? Well, actually, it's really hard to recognise because of these things. And then you get the students to experiment and push the boundaries and find out well this sort of stuff, the computer can do, this sort of stuff computer's not very good at. It's the same project, but you just you get the opportunity to dig a little deeper with some of the older students.
Nic Hughes:Think um... To sort of go back to what you said a minute ago, Carrie Anne about that... and what James said as well about younger kids, understand sorting things really well. So I think a context around what is a cat and a dog, what is a cow and a sheep, that's really it's a really simple thing that they can do. But actually, that's a nice context for them. And it of course quite funny when they are "why are you identifying this cow as a sheep or dog?" And it's then starting to have that conversation."Well it looks a bit like it because of the colour. And suddenly you're having the same conversation you've you had with the child, with the hand, and the person is a flat piece of paper, you're having that same conversation with them again, because they're starting to understand a little bit more at their level anyway, that it's not as simple as we think it is. We look at it and know what it is, a computer does not have our reasoning capabilities, does it, not quite in the same way. So therefore, our reasoning when we look at picture and go"I know what that is straight away", but a computer, it's not as simple as that. So it sort of highlights those its limitations even at a young age. So I think that's the I think that's why is very important just to talk about machine learning, because it's around us everywhere when... I don't see it going away tomorrow at any point is it? I don't think. And so, therefore, it's in the same way we're talking about programming because it's around us all the time. Machine learning is that next little bit and AI is that next little bit, the next stage of programming. So we need to have an understanding of that in just the same way. We need to have an understanding of, you know, how our banking works vs.... Oh banking's a bad example isn't it you know, how traffic lights work is a much more basic example isn't it. We need to know how light bulbs work. You know, it's a part of the world around us, as you said, it's a part of the real world, the physical, world around us. But it's that digital world as well that we're all a part of. So it's really important to have those conversations and expose our kids to them as as early as we can in the right context.
Dale Lane:And students, because I, I do a projects, and they're often surprised by what the computer can and can't or thinks that well "why can't the computer work that out? So one I did, was on recognising apples and tomatoes, pictures of apples and tomatoes. And you make sure that we train it with photos of green apples and red tomatoes. And then you show it a red apple and it thinks it's a tomato. And like you were saying Nic, we think it's so obvious " well of course, that's a tomato. Of course, that's an apple" Why can't this really smart clever technology tell what an apple is. And that's because you've told it that red things are always tomatoes. So that's what it's learnt from your training. So I really like examples where you get kids to realise that, yes, this technology is everywhere and yes, it's really powerful, but it has limitations and it's limited and affected by what we teach it.
Nic Hughes:Think I've done years ago was machine learning models and accidentally taught it left and right incorrectly. Because I don't know my left from my right. So I accidentally did it the wrong way. And it was doing it wrong, I was like "why is it doing it wrong?" And then realised, oh, I've just taught it the wrong dataset. So, of course, the idea of bias is if you teach it, incorrectly, incorrect information. You know, I've taught left right the wrong way around. And that'ssort of just really highlighted it for me years and years ago when I've used something, I can't really remember the tool I was using to do it. But it just that thing of it's those fun discoveries, isn't it, that the children get while completing the projects, which is probably almost more beneficial than the sort of the programming side of all the model building side, because I don't think in terms of the stuff we do primary definately, I don't think any of the programming elements are that complicated. And I think your website is really good at supporting and scaffolding that side of it. And I so I always think the machine learning model is the more interesting bit of it. And building that side of it, definitely at the primary age we're talking about
James Robinson:Um thinking about about training One of the things we do with this to generate our transcripts for every episode is we run our audio through a machine learning transcription service. And I still haven't got it to recognise Carrie Anne's name it constantly refers to her as"Carry On Philbin", which I'm working on correcting, but we haven't got there yet.
Carrie Anne Philbin:This idea of when to introduce and AI is really interesting. We surveyed our listeners via social media asking: "When and how do you introduce machine learning concepts with your learners?" Results have been quite mixed, but mostly they indicate that you prefer to introduce these concepts to ages 11 to 14 and 14 to 18, which I think is really interesting considering our two guests both talked about much younger age groups.
James Robinson:That is is really interesting. I think maybe there's room for these concepts to be kind of a taught a little bit further down the school. Certainly, I think the view that I guests had. Just a couple of comments that we had from our audience as well. Stuart, Cording talks about having taught machine learning with age seven plus age groups and 11 plus their local school. They run a programme on the PC, which used their camera to learn traffic light colours with a neural network, and they trained their camera and learnt how cameras can "see" in inverted commas. And we also heard from Jon Witts, who teaches machine learning and AI to his year 9 students that's age 13 to 14. And they cover it in a short unit which looks on the future of work and ethics, things like driverless cars and other technologies. And think's in that context, it might be harder for younger students access. But he's sort of he's looked at the machine learning for kid's resources and thinks, that they would be a great resource for younger learners.
Carrie Anne Philbin:Well, my thanks to Dale Lane and expertise with us today. You can read both their articles and activities in Issue 12 of Hello World magazine. If you have a question for us or a comment about our discussion today, then you can email via podcast@helloworld.cc. Or you can tweet us at helloworld_edu. So, James, what did we learn?
James Robinson:Well, I, I personally learnt, I learning and A.I. it's not beyond the reach of our youngest learners. And there are interesting and engaging ways for them to begin to understand these concepts. And, whilst, a lot of resources are becoming available. I think there's still room for some of those tools. And the ways that ways that learners access this content to kind of develop a little bit further. But it's really interesting. And how about you, Carrie Anne?
Carrie Anne Philbin:Well, I mean, I've learnt what that machines can't understand my name or transcription services always call it Carry On Philbin
James Robinson:And indeed Carry On Philbin.